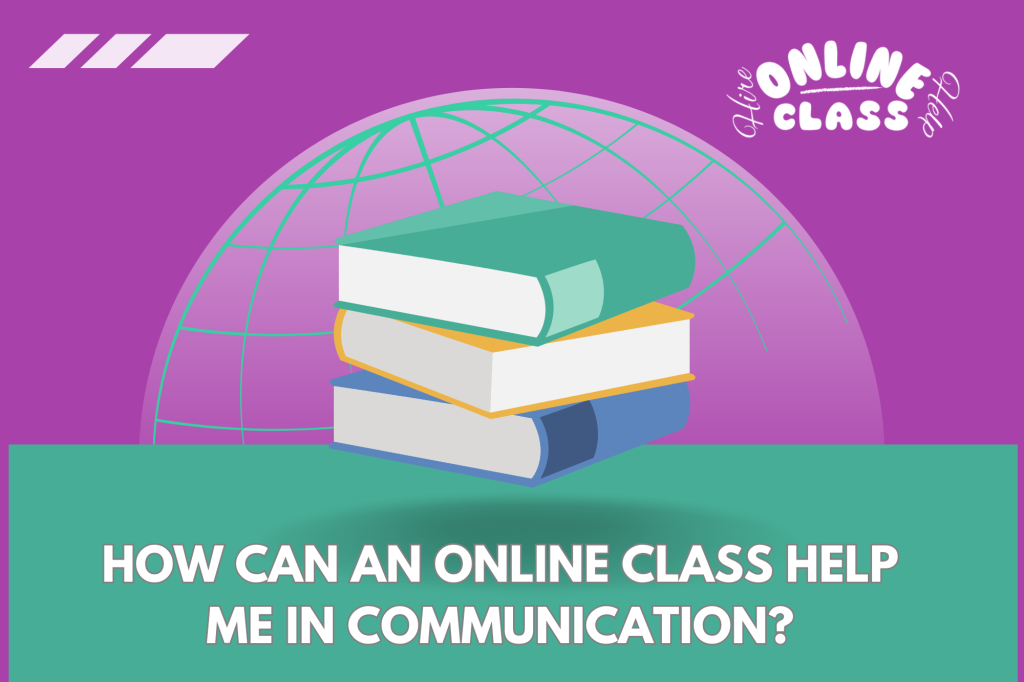
Name
Capella University
RSCH-FPX 7864 Quantitative Design and Analysis
Prof. Name
Date
Understanding the interplay between historical and contemporary performance can yield valuable insights into the stability and development of student learning. Numerous elements contribute to a student’s achievement in any specific course; however, a student’s prior grade point average (GPA) serves as a broad reflection of their academic background and abilities. In this analysis, four continuous variables are examined: Quiz 1, GPA, Final Exam, and Total points.
The descriptive statistics presented in the table below illustrate the skewness and kurtosis values for both GPA and the final exam scores. The GPA shows a skewness of -0.22 and a kurtosis of -0.69, while the final exam reveals skewness of -0.34 and kurtosis of -0.28. Since the skewness for both measures remains within the -1 to 1 range, it indicates that the distributions are largely symmetrical. Additionally, the values falling between -0.5 and 0.5 further support the notion that the distributions of GPA and final exam scores approximate a normal distribution.
Variable | Mean | Std. Deviation | Skewness | Std. Error of Skewness | Kurtosis | Std. Error of Kurtosis |
---|---|---|---|---|---|---|
GPA | 2.862 | 0.713 | -0.220 | 0.236 | -0.688 | 0.467 |
Total | 100.086 | 13.427 | -0.757 | 0.236 | 1.146 | 0.467 |
Quiz 1 | 7.467 | 2.481 | -0.851 | 0.236 | 0.162 | 0.467 |
Final | 61.838 | 7.635 | -0.341 | 0.236 | -0.277 | 0.467 |
Table 2 shows a weak positive correlation between GPA and Quiz 1, represented by a correlation coefficient (r) of 0.152. With 104 degrees of freedom (df = n-1) and a significance threshold of P=0.01, the calculated P-value is 0.212, which exceeds 0.01. The effect size is 0.152², equal to 0.023104, indicating that Quiz 1 explains only 2% of the variability in GPA. These results lack statistical significance, meaning the null hypothesis cannot be rejected.
Variable | Quiz 1 | GPA | Total | Final |
---|---|---|---|---|
Quiz 1 | Pearson’s r | — | — | — |
GPA | 0.152 | — | — | — |
Total | 0.797* | 0.318* | — | — |
Final | 0.499* | 0.379* | 0.875* | — |
p-value | 0.121 | — | < .001 | < .001 |
Conversely, the strongest correlation in the matrix is identified between the final and total variables, which demonstrate a significant linear relationship with r=0.875, a P-value of 0.000, and 104 degrees of freedom. The effect size of 0.875², amounting to 0.765625, indicates that the final exam accounts for 76% of the variation in the total score. This relationship is statistically significant with an alpha of 0.05, prompting rejection of the null hypothesis. Furthermore, a moderate linear correlation between GPA and the final score is also apparent, with r=0.379. The associated P-value of 0.000, alongside 104 degrees of freedom, indicates that the effect size of 0.379² equals 0.143641, suggesting that the final exam explains 14% of the variability in GPA. Given the significance of the findings, the null hypothesis is rejected, affirming that a substantial linear relationship exists between GPA and the final score.
While there is insufficient evidence to substantiate a significant correlation between GPA and Quiz 1 scores, significant relationships have been identified between final and total scores, as well as between GPA and final scores. The following conclusions can be drawn regarding these correlations:
Conversely, there is strong evidence for a significant relationship between final and total scores based on this dataset:
Furthermore, data supports a statistically significant linear relationship between GPA and final scores:
In the realm of veterans’ healthcare, correlation analysis serves as a powerful method for exploring the relationships between experiences during military service and the development of specific medical conditions. By rigorously examining health outcome patterns among veterans, researchers can ascertain whether certain illnesses or conditions are more prevalent within this population compared to the general public or other comparable groups. For instance, if veterans exposed to particular environments or chemicals during their service display higher incidence rates of a specific condition than those who were not exposed, a positive correlation could imply a potential service-related connection. When such correlations are robust, consistent across various studies, and control for other potential causal factors, it strengthens the argument for designating these conditions as “presumptive.” Recognizing conditions as presumptive streamlines the process for affected veterans to secure benefits and treatment, as they are no longer required to provide proof that their condition is directly linked to their military service. Instead, the service connection is presumed based on the statistical relationships established through comprehensive research.
Betancourt, J. A., Granados, P. S., Pacheco, G. J., Reagan, J., Shanmugam, R., Topinka, J. B., Beauvais, B. M., Ramamonjiarivelo, Z. H., & Fulton, L. V. (2021). Exploring health outcomes for U.S. veterans compared to non-veterans from 2003 to 2019. Healthcare (Basel, Switzerland), 9(5), 604. https://doi.org/10.3390/healthcare9050604
Creswell, J. W. (2014). Research design: Qualitative, quantitative, and mixed methods approaches (4th ed.). SAGE Publications.
Field, A. (2018). Discovering statistics using IBM SPSS statistics (5th ed.). SAGE.
Gravetter, F. J., & Wallnau, L. B. (2016). Statistics for the behavioral sciences (10th ed.). Cengage Learning.
McHugh, M. L. (2013). The Chi-square test of independence. Biochemia Medica, 23(2), 143-149.
Get In touch
Let's Connect: We're Here to Help You Succeed!
Have a question or need support? Connect with our team today. We’re ready to assist you with personalized guidance to help you achieve your academic goals. Reach out via email, phone, or our easy-to-use contact form.
For urgent help
+1 (571) 899-4759
Mail us 24/7
info@hireonlineclasshelp.com
Get expert assistance to excel in your courses with personalized support. Our creative approach ensures your academic success every step of the way.
Our Services
Copyright © 2024 hireclassonlinehelp.com All Rights Reserved.