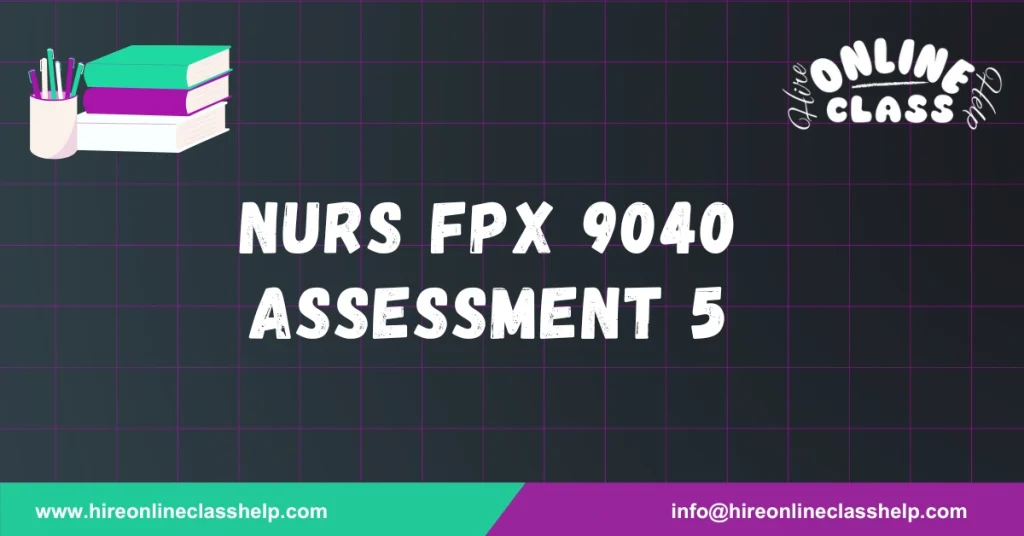
Name
Capella University
RSCH-FPX 7864 Quantitative Design and Analysis
Prof. Name
Date
In the independent T-test, the variables involved are gender, which serves as the independent variable, and GPA, which is the dependent variable. Gender is a categorical variable, while GPA is continuous. The research question guiding this analysis is: Does gender influence GPA levels? The null hypothesis posits that gender does not impact GPA levels, implying that the mean scores within the sample are not significantly different. Conversely, the alternative hypothesis suggests that gender has an effect on GPA, indicating that the mean GPA for females and males differs significantly.
Levene’s Test for Equality of Variances | t-test for Equality of Means | ||||||
---|---|---|---|---|---|---|---|
F | Sig. | t | df | Sig. (2-tailed) | Mean Difference | Std. Error Difference | 95% Confidence Interval of the Difference |
———- | —— | ——- | —– | —————– | —————- | ———————- | ——————————————- |
0.095 | 0.758 | 1.999 | 103 | 0.048 | 0.28090 | 0.14055 | Lower: 0.00215, Upper: 0.55965 |
1.961 | 79.985 | 0.053 | 0.28090 | 0.14326 | Lower: -0.00419, Upper: 0.56599 |
The results from Levene’s test for the equality of variances (Levene, 1960) are examined to determine the homogeneity of variances between the dependent variable, GPA, and the independent variable, gender. The significance value obtained from Levene’s test is analyzed to check for any notable differences. A significance level below .05 would indicate that the variances are significantly different, whereas a significance level above .05 suggests that the variances are approximately equal. In this case, the reported significance level between GPA and gender is .758, which exceeds the threshold of p=.05. Therefore, equal variances are assumed, indicating that the assumption of homogeneity has been satisfied.
Gender | N | Mean | Std. Deviation | Std. Error Mean |
---|---|---|---|---|
Female | 64 | 2.9719 | 0.67822 | 0.08478 |
Male | 41 | 2.6910 | 0.73942 | 0.11548 |
The statistics concerning gender and GPA are presented in Table 2. Females (n=64) have a mean GPA of 2.97, with a standard deviation of 0.678, while males (n=41) show a mean GPA of 2.69 and a standard deviation of 0.739. Based on Levene’s test, equal variances are assumed, and the assumption of homogeneity has been satisfied. The calculated significance (2-tailed) value is 0.048. Since 0.048 is less than p=0.05, it indicates a slight difference between the variances for GPA and gender, although it fails to reject the null hypothesis. This suggests that the variability between the groups is not significantly different, indicating that male GPAs are lower when compared to female GPAs.
This analysis explored the GPA differences between genders (male and female). An equal variance t-test revealed a statistical disparity between the mean GPAs. The data gathered included a total of (N=105) students, consisting of both males and females. The results indicated that females had a mean GPA of M = 2.97 (SD = 0.68) while males had a mean GPA of M = 2.69 (SD = 0.74). The t-test was executed at a significance level of P=0.05, yielding F (1,103) = 0.95, p = .758 > 0.05, leading to the conclusion that gender does indeed influence GPA. However, several limitations were noted. Field (2018) points out that violations of assumptions related to the between-samples t-test may affect results. Additionally, the unequal sample sizes may skew results, with females being N=64 and males being N=41. Such disparities can contribute to higher GPAs among females. Future studies should focus on identifying effective support networks that enhance GPA achievement for students.
An effective HR leader must consistently analyze trends that may affect the workplace. Such trends might include time and attendance, staff turnover, and benefits utilization, among others. Human Resources can utilize these insights to identify factors influencing employee performance and satisfaction, allowing them to assess the elements that contribute to different outcomes. For example, one could compare staffing initiatives against new hires, treated as the dependent variable, or examine the impact of injury and safety training programs.
Field, A. (2018). Discovering Statistics Using IBM SPSS Statistics. SAGE Publications.
Levene, H. (1960). In Contributions to Probability and Statistics: Essays in Honor of Harold Hotelling (I. Olkin et al. eds., pp. 278-292). Stanford University Press.
Get In touch
Let's Connect: We're Here to Help You Succeed!
Have a question or need support? Connect with our team today. We’re ready to assist you with personalized guidance to help you achieve your academic goals. Reach out via email, phone, or our easy-to-use contact form.
For urgent help
+1 (571) 899-4759
Mail us 24/7
info@hireonlineclasshelp.com
Get expert assistance to excel in your courses with personalized support. Our creative approach ensures your academic success every step of the way.
Our Services
Copyright © 2024 hireclassonlinehelp.com All Rights Reserved.